Beyond the Code in AI Careers: A Gen Z Perspective
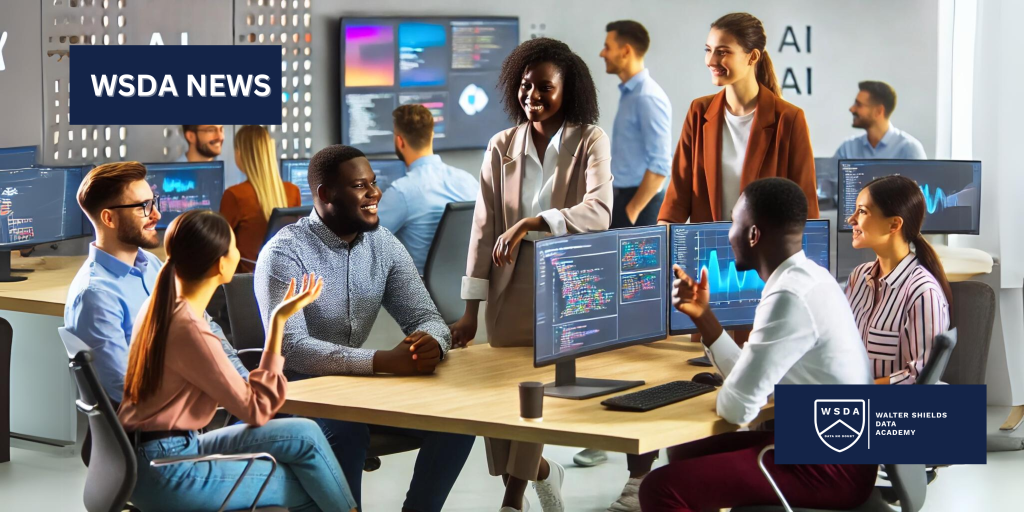
In the world of AI, there’s more than meets the eye beyond just coding. Take it from Pranjali Ajay Parse, a 25-year-old data scientist at Autodesk, who offers a perspective that challenges the typical assumptions about AI roles. She’s been developing an AI tool that provides employees with insights into their work patterns, such as meeting trends and routines. Through her experiences, Parse has come to understand that working in AI involves much more than just writing code.
The Multifaceted Nature of AI Roles
Many might think that a strong command of Python is sufficient for landing an AI position. However, Parse explains that AI is inherently interdisciplinary. It borrows from mathematics, computer science, statistics, and domain-specific knowledge. While a formal degree in AI isn’t mandatory, skills like case study analysis, SQL querying, and coding are crucial. Boot camps and personal projects can be great ways to gain these skills.
Parse shares that about 70% of her role revolves around data science, involving the review and analysis of data sets. The rest of her time is dedicated to software engineering, building data pipelines, architectural design, and plenty of mathematics. Staying updated with advancements in related fields is also vital, given the rapid pace of technological evolution.
Collaboration is Key in AI Projects
The stereotype of the solitary software engineer doesn’t apply here. According to Parse, AI projects are rarely solo endeavors. The collaborative nature of AI necessitates close interaction with multiple teams and stakeholders. In her work on an AI recommendation system, she collaborates with seven to eight different teams.
The process starts with data collection and preparation by a data analysis team. Next, data scientists use statistical methods and modeling techniques. The machine learning team develops and refines the model, while UX and UI experts design the user interface. Software engineers then build the front end, and finally, the marketing team strategizes the product’s launch. An end-to-end AI project demands substantial communication and teamwork.
Ethical Considerations in AI Development
Ethics play a significant role when dealing with sensitive data during AI development. Privacy protocols are extensive, requiring permission for tasks involving personal data. Measures like pseudonymizing identities and preventing biases are crucial in ensuring ethical AI outcomes.
Compliance with legal and regulatory requirements is a must, as is considering projects’ long-term implications. This involves pondering potential unintended consequences and ethical dilemmas. While privacy may seem obvious, Parse notes it’s easy to get caught up in model performance and overlook overarching implications. Companies must train employees on privacy and ethical guidelines, but individuals should also adopt a broader perspective on their work.
In closing, AI careers demand more than technical prowess. They require collaboration, multidisciplinary knowledge, and ethical awareness, making them challenging yet rewarding pursuits for aspiring professionals.
Data No Doubt! Check out WSDALearning.ai and start learning Data Analytics and Data Science Today!